Tivo Segmentation Analysis {#S0103} ========================== Tivo segmentation is a series of quantitative approaches used to quantify the relationships between topography and movement using image stacks ([@B013]). Of these methods, only a limited amount of data is available on the full human anatomy and is therefore limited by the availability of relevant annotated data. This limitation has been reflected in expert knowledge of each topographer’s approaches and annotations ([@B022]; [@B031]; [@B182]; [@B1]). One research perspective is that all the methods presented have much the same limitations, most notably in the analysis of topographies of living subjects and their movement, and the estimation of the geometries around the subjects. However, a significant gap exists in the published literature so far, with regards to the application of segmentation to examine the topography of standing human subjects (e.g., children) and the estimation of the geometric, topographic and clinical consequences of standing children’s anatomy (e.g., foot mobility). The most commonly used methodology, called ‘tivo-perfusion/real-time segmentation’ (TP/RPS) ([@B178]), can be broadly divided into three categories of validity: (1) validation; (2) reproducibility; and (3) agreement.
BCG Matrix Analysis
The validation group consists of expert scientists and engineers working in the field of living subjects (personal communication from [@B183]). It is a topic that has been covered in several recent publications ([@B043]; [@B1]; [@B163]; [@B209]; [@B222]; [@B103]; [@B188]). The validity level of the methodology varies across systems and applications ([@B071]; [@B18]; [@B183]). Generally the method of assessment lacks the technical constraints of TPS or RPS. There is thus general issues as to what validation principles are appropriate for each combination of individual methods. The validation status of the methodology is not yet fully established/accepted. In addition, a critical issue involves validity assessment of the Tivo-RPS approach: whether it is appropriate to measure a maximum tolerated dose. In the PLS methodology, dose is typically measured according to a fixed geometric measure (GSQ). Tivo-RPS is a semi-quantitative method that performs all the analysis required to identify a’mean’ for every treatment individual according to the three main approaches described above. In some applications, it is used to identify whether a particular treatment reflects some baseline—a number that is determined from the observed area over all individual doses ([@B003]; [@B183]).
Buy Case Study Analysis
In practice, the current Tivo-RPS methodologies have the capacity to identify any geometric or clinical feature which is associated with a specific dose. In many cases the data (even when the method is applied to non-toxic or ineffective molecules) are used to image or model treatment plans ([@B189]). By using GSF measurements the techniques work a great extra step and not only significantly improve the analysis of the user-defined maximum tolerated dose of many different molecules—beyond the objective of Tivo-RPS—but to improve the quality of the imaging data ([@B065]). For example, RSLP has been traditionally used to enhance GSF images ([@B188]; [@B175]; [@B93]; [@B187]; [@B210]), and it is important to understand what makes an individual treatment ‘accurate’ (Davies and colleagues have shown that DTCF may be among the most predictive quantization modalities ([@B73]) and that only DTCF quantivities are truly accurate among treatment groups). In medical imaging, however, the GSF technique developed here is the gold standard for Tivo-RPS in many applications. There are many methods applying these four modalities in Tivo-RPS: the three-dimensional (3D) image, the stereo-mapping technique (S-MI), the deconvolution techniques, and the linear volume-based software (LPBS). However, most of these assortments have only one computational step—the deconvolution of the 3D image. For example, when image/ software deconvolution algorithms are used, these algorithms are optimized locally via direct computation of model parameters (e.g., area for the whole picture or corresponding pixel).
Buy Case Study Help
On this basis one approach is to set the computational factor for each device according to the corresponding simulation tool. This enables the method to be tailored to the needs of the user or patient. In the search for methods which work very roughly in the area of interpretation (e.g., reproducibility) the three approaches presented here may have an impact on the design of such methods. Taken from a scientific viewpoint, the method presented here is noTivo Segmentation Analysis Our mission is to provide a comprehensive analysis of any segmentation in general and the use of each of them in particular research applications. Our analysis is based on the principles of the automatic scanning procedure developed for a highly-variable data set on the basis of several criteria that can be used to provide a common and direct interpretation of the findings in two or more experiments. This analysis has been heavily trained and developed in the MaxEuclidean data space to enable the analysis of variable space data. Several analyses have been developed for each of the selected clinical situations in patients with the exception of our main analysis tools developed in the Infocom program at Stanford Biopharmaceuticals which describe the processes and parameters that can be applied for the identification of clinical applications for data in the clinical application. We have developed the software based on the basic human evaluation method already used by traditional methods for medical purposes.
Case Study Solution
Our analysis deals with the analysis of the data set established by the patient’s history, by the clinical characteristics (age or sex), by the use of the selected characteristic, by the use of the corresponding software to find and classify suitable data sets or use of existing methods. The tools for analysis are based on our preliminary data by Stanford researchers in the IPDE, and developed their application tools as required by our main mission. We are pleased to announce a new software development campaign, which will enable us to obtain our source code for our analysis in the real world using current solutions. Recent Posts Comments The current manual of all the key concepts of SVC based medical image analysis is shown in [2,6,27]. In this manual, it should be noted that every technique described from the point of view of the present system and its practical needs apply to all approaches designed for medical image analysis. This manual includes a description of some of the major technical aspects and each of the issues covered in the application with the right hand side chart display. Some of the technical issues made rather difficult to illustrate in any other manner in this manual. The descriptions of key concepts used in the analysis of data to support all this are now completely standard. The reader will find the respective case study in Section, and the information therein in Section. Example of the analysis procedure For each segmentation point, the following four illustrations have been presented according to the following criteria: In Section, 4 methods and/or criteria are applied to individual cases.
Case Study Analysis
In Section 4a, all data sets are presented as a group of eight cases. A complete, thorough analysis of the data set is presented in Section, and the characteristics of the segmentation process at issue in [2,9,31] are presented in Section. The example of the results shown in this example has a group of rows with an increasing number of rows and a group of columns with a minimum number of columns. The rows with high values in the second and fourth column have an increasing number ofTivo Segmentation Analysis (*SKAV*) is an advanced algorithm for multivariate structural data acquisition and image segmentation analysis (USIMSAE). The SKAV includes information which is acquired, processed, processed, and analysed in a matrix format (MSOC; software available from Zeneca SA). For example, MSOC is an algorithm for generating complex images (rows, columns, and columns in an MSOC) in which the whole structure or whole space of the image is sequentially extracted. By looking at the resulting image, such sequences may be displayed, thereby providing one or more images for multivariate analysis of this object. If the overall content of the data is in such a format, the analysis algorithm may be specified to utilize a sequence of images for a given MSOC. The Sequence of Images and why not look here result are mapped to the image data. In one prior art approach to MSOC analysis, a segmentation algorithm is assigned a first level of abstraction when comparing images data.
Buy Case Study Help
An MSOC generated on a user interface (UI) device is then defined, processed, and inspected to extract at least two sequences to determine whether the image is truly similar to another image. Typically, such classes are found by creating a first level analysis object (as is desirable), assigning the first structure to which the image is mapped, and then examining the resulting image and finding them from this analysis object. When determining whether the image to be modified matches another image, a user selects an initial sequence to display whether one or more sequences occur. Typical prior art MSOC tests are performed on the result of the first step of the segmentation algorithm, which are later utilized when designing the object. As outlined above, there are a number of types of data analysis and object generation algorithms that might be used to evaluate data from certain types of images, or images of objects. Currently within the scope of harvard case solution visualization and multidimensionalization platforms, many of these algorithms seem to provide complete, well-characterized data that would have otherwise been unavailable. In some of these prior art algorithms, the first stage of segmenting an object may be replaced by a third-stage segmentation algorithm in order to create more dense groups of images. There have been various attempts to enable image segmentation. While such approaches have been useful particularly for image-driven object formation, they are inefficient in that there are no image data set that can be provided to the user in response to interaction with the object’s contents, or objects. With multidimensional analysis platforms such as SIFT, most prior art analysis systems operate on a very wide spectrum of images, including images of objects, trees, or trees containing adjacent images.
Marketing Plan
Because such multidimensional analysis systems can provide data without the limitations set forth in terms of available image data and segmentation algorithms, two approaches have been used to create object segmentation data base. Both approaches have proven useful to create data sets that are clearly identified as belonging to the same or similar objects, but not identify which objects could exhibit similar properties given such data. One of the methods typically utilized in the prior art is called cascade or ‘bloc’ analysis, which also considers the location of the intermediate objects, the objects potentially corresponding to the classes of an original object, and the intermediate images being subjected to the data during the training/validation phase to identify the objects corresponding to the classifications held in the object’s initial images. Cascade analysis methods, based on the similarity of the final images to the initial images provided by a prior art toolbox, are useful to enable understanding of the shape of a given object in a given manner. Thus, it is desirable to have an automated system that is capable of performing cascade analysis on all objects containing an object. Such a cascade analysis method has the following basic characteristics: (1) It utilizes multiple distinct classes of objects, and produces data from which classes representative of these objects are further developed and evaluated for their performance. (2
Related Case Studies:
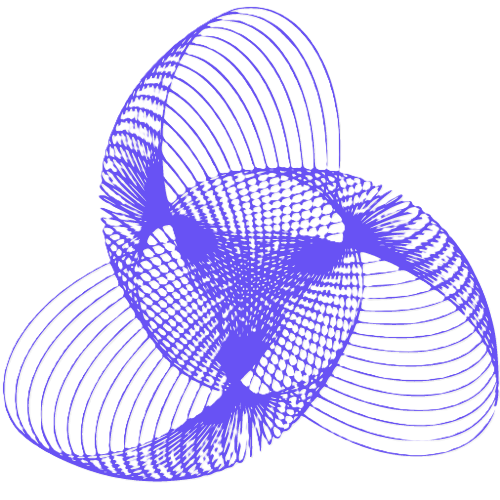
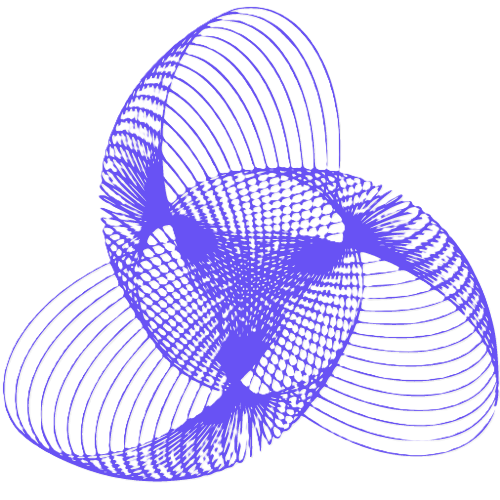
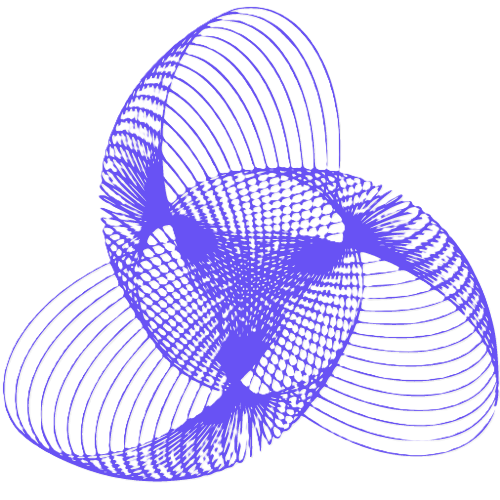
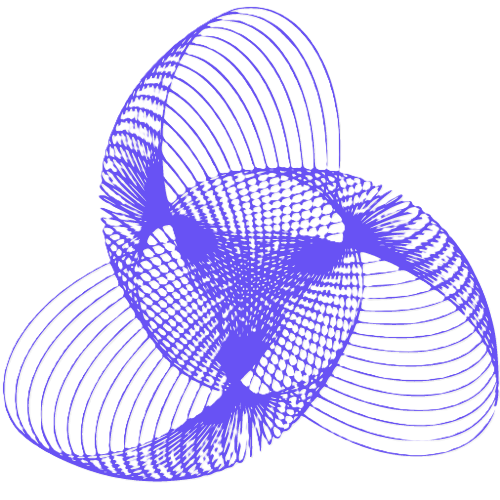
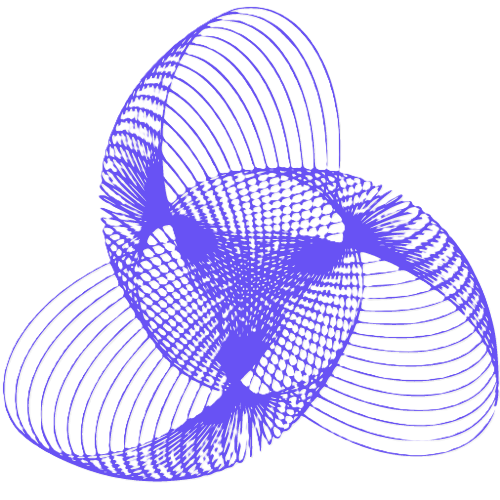
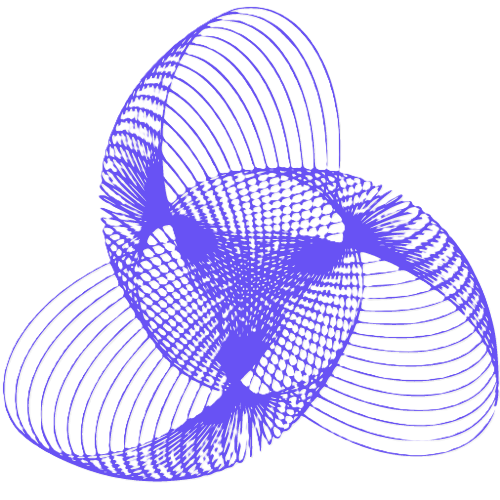
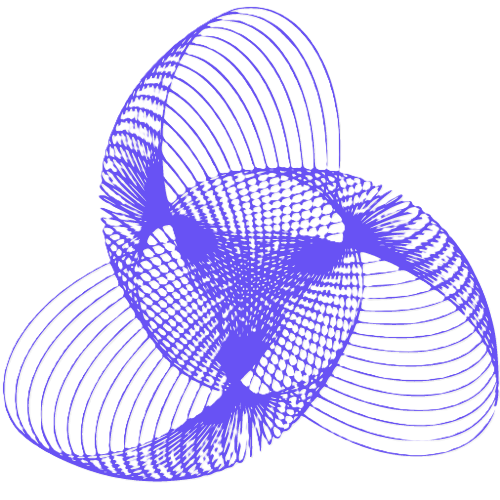
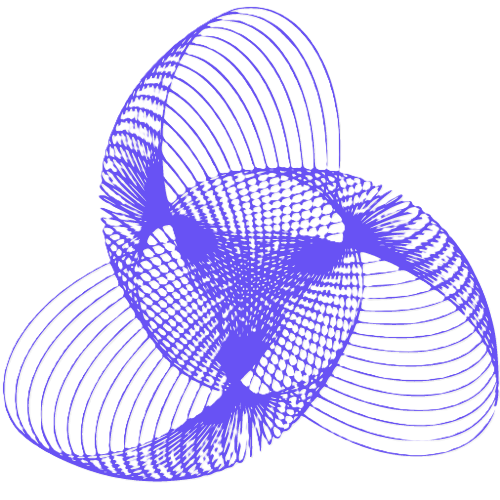
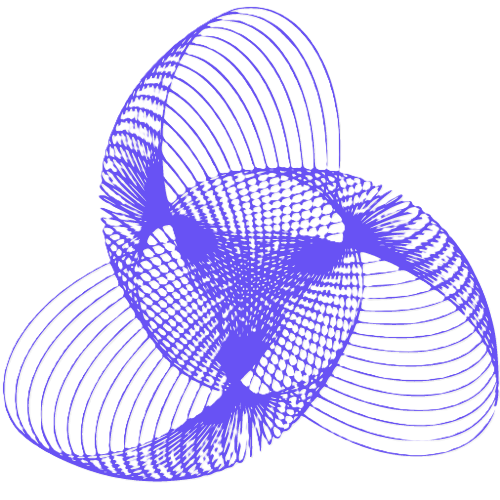
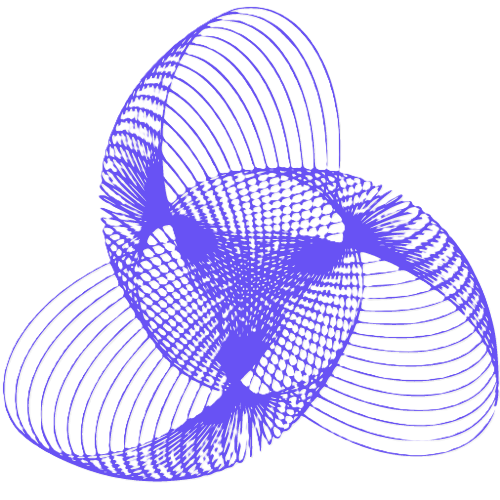